From 3D to 4D: The Evolution of Seismic Data with Deborah Sacrey
0:00 Welcome back to another energy one-on-one episode. You are here with three of the women from Digital Wildcatters. This is your one stop shop for all the dumb questions that you may be afraid to ask
0:11 about the energy industry. I am Sydney. I am joined by Misty and Julie. And today's guest of honor is Deborah Seykri from Auburn Energy. Welcome, Deborah. Thank you. Yeah. Do you want to give
0:22 us a little rundown of your background and what you do for Auburn? Um, well, I am Auburn. It's a company of one. Perfect I have been out of my own in consulting in the oil industry for over 30
0:33 years. Wow. But I went to the University of Oklahoma, boomers sooner. I thought you were going to say Auburn. Everyone thinks it's for hair color. And I know you got it. Surely, but surely I'm
0:45 going pink instead of Auburn, but that's okay. I get re-aubered next week. Um, I went to the University of Oklahoma and got a degree in geology and promptly went out and started working for a
0:55 large major at the time, which was Gulf oil in the late 70s. do the math. I just turned 70 years old. And since then I've worked for major companies, minor companies, small companies. And
1:10 finally I just said to heck with it and I went out on my own. Good for you. And I started Oklahoma City, did a stint in Dallas and then moved my way down to Houston. We just visited Oklahoma City
1:19 for the first time last week for one of our events and it was lovely. We had the best time. Now you probably went to Bricktown, right? Did you go to Bricktown while you were or in downtown? Were
1:31 you in downtown? A little bit. So our event was in Uptown, I think, kind of like a little quirky area. It was great. We stayed nearby and then we kind of bopped around town when we had some
1:42 downtime. But all of us were very pleasantly surprised about how great it was and now we all can't wait to go back. Well, I'll be going in October because I'm giving a talk at a convention up there.
1:54 Nice. So on Machine Learning, gosh Perfect, so you know it's it's been one of those ups and downs, the industry has good points and bad points, but I've survived. And about seven years ago,
2:06 well, we built a house out in the country and I put my office out there in the house. We moved three days before Hurricane Harvey. Oh, wow. That was real fun. Yeah. So. But that's - Were you
2:19 out? So you were out of Houston. We were in Maine area. But we had four moving trucks, 450 boxes, a piano mover, and they were checking the generator literally when it started raining. Oh, my
2:32 God. Oh, my God. So, fortunately the house we had here in town didn't flood. Good, good, good, good. People were lucky. Yeah. But, man, we had to come back into the office. We'd been in
2:42 the same office space. My husband and I shared an office and we haven't killed each other yet, which is pretty amazing. It is amazing. But we had some stuff left in the office and we were making
2:50 trips back and forth to pull out the last of the stuff in the office and it was pretty grim. Wow, I bet. Oh, but, you know, I love what I do, so - Well, then let's dive into it. Yeah, so
3:01 let's start with, first of all, the first question, an introduction to seismic data. What is it? And why is it important to energy? Well, that's a really good question. So basically, seismic
3:19 data is what geologists and geophysicists use to image the Earth. And what they do is it's basically sound waves that they send into the Earth that bounce off different layers of rock. And if it's
3:36 really hard rock, it bounces and gives you one kind of signal. And if it's really soft rock, it kind of slowly bounces and gives you another kind of signal. But it's when they accumulate all those
3:46 signals and put them back together processing that you have a visual image of the layers of rock in the earth. Kind of like sonar would be bouncing off the things in the ocean. The sound waves, the
3:60 energy that they put into the earth and they can do it through different means. In the ocean, they use what they call air guns, which send a big booming kind of reflection down into the ocean. On
4:12 land, they use big, vibrousized trucks that have a plate that goes down on the ground and sends a big vibration into the ground. And then they can use dynamite. They drill little holes, they
4:24 throw dynamite charges in and blow it up and then that sends a signal down into the ground. So there's many different ways of collecting the information. Is that wire line, like does wire line
4:35 usually send that? No. Who gets the seismic data? No. That's completely different. We have, well, there's power sources and then they have receivers. Got it. So they put these out in an array
4:47 on the ground and the power source of course sends the signal then to the ground and the receivers pick up the signal as it's coming back out. Okay and it comes out in waves. because it's going
4:59 deeper and deeper and deeper and bouncing off different layers of rock. So they're constantly recording and picking it all up, much like when you see an earthquake, the little needle that goes back
5:09 and forth. Well, this is a sound recorder that's picking up vibrations coming back from Europe and it's processed. And then it comes out, what in the old days, it came out on paper. And we'd
5:20 have to look at all these little wiggly lines. It's like another link. Like another link, right? No, like an EKG machine, right? It would be very similar Yeah, or the earthquake things. But
5:29 imagine if you're recording an earthquake and it just keeps happening and happening and happening, that's what we would look at. And we'd interpret that and understand based on wells that have been
5:41 drilled, we could tie back and correlate the signals to specific formations in the earth. And that's how we were able to map the subsurface. So without seismic data, We really would have stopped.
5:57 lining a lot of oil and gas years and years and years ago. That was gonna be my question. So typically the importance of using seismic data is to traditionally to find where oil is. Right, oil and
6:11 gas. Great. And you know, in the state of Texas, there's been somewhere over two million wells drilled. Geez. But the state of Texas is so big, there are huge expanses where there's no well
6:21 information. So the seismic is basically your interpolation between well control, which is your hard data. You know what's in the well, because you've got the layers of rock, but you are having
6:34 to guess what's happening between this well and this well and this well. And seismic helps us do that. Great. So you usually start the process of drilling with seismic data. Like you have to have
6:45 that first layer, going to a geologist, tell me what's underground. Right, well, you know, and that's where the roles between geologists and geophysicists
6:58 Before seismic, it was the geologist who was doing the mapping, who was interpolating what they were seeing in the wells, okay? When seismic came along, the geophysicist, which is the person who
7:11 learned how to read all the signals, started making their version of maps. And, of course, when you send a signal into the earth that comes back, they measure it in time, wells are measured in
7:22 depth. So, for a long
7:26 time, it was hard for them to take the time maps and make sense of them to correlate to the depth maps. And so, over the years, the geologist's role and the geophysicist's role have kind of
7:38 emerged. Where now, we just kind of use the term geoscientist to combine both of them. My degree may be in geology, but most of my career, I've worked in the geophysical side, right,
7:49 interpreting the seismic data. This is perfect timing because we have a podcast right after this that is more of a general geology. science 101. So these are going to be perfectly paired. So I
8:03 feel like this might be our first dumb question. When you're capturing seismic data, what exactly goes into the earth?
8:12 Is it? I'm pitching it like a laser? No, it's,
8:16 you know, it's like sonar. So it's sound? Or like vibration? Yes, that's it. Is it a big machine? Well, that's where the vibraside machine or the dynamite. Okay. And in my world, there are
8:29 no dumb questions or only non-answers. So feel like that you can ask me anything. And if I'll let you know if I think it's a dumb answer Maybe we could find a good graphic of what the machine looks
8:42 like. Oh, yeah. So they roll it out and turn around and turn around. It's a big truck. Okay. It's a huge massive truck that probably weighs 40, 000 pounds. Wow. And they put this big thick
8:54 steel middle plate. on the ground to the extent that
8:60 it pulls the truck up. It's literally supporting itself on this flight so that they have all the weight in the force of that truck when it sends its signal and it sends out
9:11 signals in decibels or in Hertz. Much like what you guys are doing in recording and you're looking at the sound. You know, you know the decibels, you know the Hertz. It's that kind of information
9:22 that's going into the ground and coming out and being recorded. And they put the recordings on these massive tapes.
9:30 Much like a videotape would be. And then they process it. Yeah. And the processing is what brings the squiggles to light. So in
9:42 the past, like what are some of the common challenges besides like the geophysicist, geologist, like that misinterpretation of the different types of data? Are there any other common, like?
9:55 struggles whenever you're trying to read the data or gather the data and It's very interesting that you've been in the industry since the 70s because I'm sure it's changed a lot So massive it'd be
10:08 interesting to go back into the past and like what was it like then like how hard was it? You know, it's one of those things that as you get older you look at someone and you say I never want to be
10:18 that age again
10:21 I never want to go back What we had to do right when we were when we were working, you know in the early in the 70s and 80s the one of the biggest things and and which really revolutionized the
10:35 industry is When they first started acquiring seismic they only acquired it along a straight line They would put rows of they would put receivers and and out and power sources out and they collected
10:49 it in one straight line Interesting, which is good Unless you want to know what's happening. on this side of the line, what's on the side of the line? Yeah, right. But one more ground together.
10:58 Yeah, one more ground together. And so in the, I wanna say about the mid eight, the big company like Exxon and Chevron, started experimenting with what would happen if we laid rows and rows and
11:11 rows and rows of straight line out and then processed it all together. And that became three dimensional sides of me. So now you're not looking at just the slice of the earth You're looking at a
11:23 cube of the earth. And that helped us, I mean, it just revolutionized and changed the industry all over the world when we could see now in three dimensions instead of two dimensions. That seems
11:37 like it was a huge deal. Yeah, it's a darn big deal. And then in the 90s, so at first only the big companies could afford to do that. So they held all the licenses for the big 3D's they were
11:49 shooting, including Marine And then in the '90s, the - Technology improves so much that the little guys started getting all the 3D and doing their own thing. And so now, when you're putting a
12:03 prospect together and you're trying to convince people that you wanna drill a well, if you basically don't have seismic to show or a 3D on how you triangulated all the well information in, then
12:15 you're not gonna be able to get your well drilled. Right. 'Cause everyone's used to that technology now. Exactly Yeah, that kind of leads into kind of the second part of our discussion in seismic
12:27 data, and now that we're kind of in this digital age, you touched on it before how much like an E, what's it called? An EKG? Artificial. Isn't EKG the heart monitor? Yeah. So much like an EKG
12:40 machine, I'm picturing it back in the old days, printing out paper, just a ton of lines that someone would physically have. So nowadays I'm assuming it is all, digital? On a digital, on the
12:52 computer, you know, I've got three screens, it looks like the control board to the USS Enterprise in my office.
12:59 But you know, and one reason why I do bifocals instead of where they transition is because when you don't when you have the transitional ones, you lose your peripheral vision. So bifocals, because
13:12 I'm looking at what's going on this screen, what's going on this screen, what's going on this screen. I like to keep my peripheral vision. Right. Yes. Precise as I can. I just. A little nuance
13:21 to throw in there. But no, it's all digital and you sit there in front of a workstation and look at squiggles all day long. Color. I like to look mine in color. And that's where the machine
13:34 learning part of us come in, because the machine learning is definitely in color, because you're picking up all these different patterns in the earth and you want your eyes to pick up on unique
13:44 patterns. So it's all in color. So speaking of machine learning, before we dive into how seismic data is leaning into machine learning now, you gave us a great example of what machine learning is.
13:58 Can you repeat that? Well, you know, we live with artificial intelligence, all of our, I mean, now, every day. And a good example of,
14:08 okay, so machine learning, you have a training data set that the machine learns from, okay? And then when it learns from that training data set, it can go out and do different things to enhance
14:22 that training data set. So a good example that I mentioned earlier is you buy something on Amazon, okay? And so later on, you're cruising through the internet and a pop-up ad comes in and gosh,
14:35 it's the thing that you just bought. Or people who bought this also bought this, okay? So you become the training data set. Those get me every time you become the training data set for Amazon.
14:48 They're learning what you like, what you're, you like color-wise or what you like cooking-wise. I mean, I make a mistake of going to all-clad and buying a pot. The next thing I know, every
14:59 cooking device in the world comes up on my own here. So that's a really good example of how our everyday lives are affected by machine learning and artificial intelligence. So now we're taking that
15:11 training data set and making a 3D, the training data set, and having the machine go in and see things inside that seismic data that our eyes and our brains are not picking up. Right. And that's a
15:25 huge enhancement, another great technology change. When did that start shifting? Gosh, well, of course, machine learning has been around for a long time and AI has been around a long time,
15:37 especially in the medical industry or Wall Street, pattern recognition, cluster analysis, all that kind of stuff has been there for a long time It really has only been applied Um
15:51 to the extent that we're using it now in the seismic data industry, probably for the last 15 years. Okay. And it's being slow to catch on because people are going, well, I've been doing it this
16:04 way for 40 years. I'm not sure I want to switch over and do it this way. I'm sure the people that were reading the physical papers said the same thing when it was all going. Yeah, most assuredly.
16:16 So it's been a slow to adopt kind of technology But in my world, or what I say to people, as I say, look, all the easy stuff has been found. We've got seismic, literally covering the world. And
16:30 people have hammered over the same 3Ds for 20 years, trying in their brains to see something new. You're gonna have to apply new technology to be able to see anything new. Right. And that's -
16:44 That's a good point. That is a good point. I mean, that's where we're coming. Yeah
16:49 And a lot of people ask me, Well. you know, if machine learning is so good and it's helping you find the oil and gas that much easier, will it replace the geologist or geophysicists? And a lot of
16:60 people can be replaced by machine learning. I mean, look at robotics and all the automation they're doing in the oil field. And that would be another really good podcast for you guys. But you
17:11 still have to interpret what the computer gives you.
17:16 And so you have to have a knowledge of the subsurface, which the machines don't, they're just giving you colors and patterns. They don't know what it means. You have to understand what it means.
17:25 And you're tying into all the well information too. So you still have the upper hand when it comes to the machine learning in the seismic world. I feel like we hear that a lot right now with AI. AI
17:34 has just hit a huge bubble like everyone's talking about AI. And everybody's worried about their jobs getting taken. But I look at it as enhancing. You can put out so much more with the help of AI.
17:49 And I'm sure it's the same way. your job better and more efficiently so you have other, you have more time to do other things. Well, when the computer is cranking on something in my office, I go
17:59 in and put on a pot of soup. I mean, yeah, we're multitasking. So no, no, you're totally right there. But, but again, the machine doesn't know anything except what it's coming back with
18:10 information. And it's up to the humans to understand what that information means and how to apply it Right. Are there challenges with the accuracy of it? Or once it kind of gets rolling and it's
18:24 testing the test group of the data is there. Obviously, I'm assuming the person on the other side that's interpreting it is giving feedback, hey, this might not be accurate. But are there ever
18:34 concerns with that? How, how was that proactively sort of looking for? I'm assuming when they give the training data set, is it already accurate? So
18:48 reliable. Yeah, that's that's a that's a real Um, because, and, and I'll show this in some of my slides and the PowerPoint later on, but, but so the, the stuff that I'm doing, I'm looking at
19:02 timing. Okay. So seismic data comes back to you in time. How much time does it take to go down to this reflector and bounce back? Okay So for 60 years, we have been interpreting in the time
19:16 domain and And it's been okay. You have you have a wiggle that goes this way Which means a hard rock and a wiggle that goes this way which means a soft rock and a wiggle that goes this way But we
19:29 know that there's a lot of stuff happening between the two pieces of hard rock And now with the machine learning we're breaking it down into smaller and smaller pieces Statistically the computer can
19:43 do that our eyes cannot right so now we're seeing finer and finer detail that we've never been able to image before And so the the problems arise with the well calibration. Okay? When when you're
19:58 looking at just a wiggle going this way and a wiggle going this way and a wiggle going this way and you've got a Well right here, you've got a lot of slop Right, but when you start breaking down
20:08 mother nature into very concise beds Then then the tie of the time to the depth has to be very accurate Mm-hmm and if you're if you're not tied to the well accurately Then you can misinterpret what
20:23 the patterns mean and and and lose What could be a good oil and gas opportunity into something that may be a wet sand or something like that? So right so the critical point when you're when you're
20:36 dividing the time up into smaller increments Statistically is is how accurate are your well ties right and and the change of rocks the change from say sandstone to shale to limestone
20:51 All create what they call velocity variances in the ground because the signal will go through a limestone Much faster than it will go through a gas prone sandstone interesting. Okay, so a good way
21:05 to test how we see gas Okay, the next time you have a chance get a really nice crystal glass and fill it with water and Then use a fork on it to hear that's the sound Okay, then drop an alka seltzer
21:22 in there give it about 15 or 20 seconds and then hit that thing again And it'll go that that that it should do that. We should you know, it's very it's a very I do this You know for when I when I
21:33 speak it like junior highs or for kids or something like that like for us right now But but but it's and I had I was doing that I was doing that experiment one time and a guy in the audience and said
21:45 Well, that's how I tell my scotch and soda from my scotch and water I'm going, Well, I'm your party with scotch. You know, Alka Seltzer works really great. But it changes the sound in the rock.
21:58 And that's how we interpret them. That is very interpret them. When it's processed and comes back to us, we can see that change in velocity. We can see that change from the ting to the thud,
22:10 right? The difference between a wet sand and a gas sand. I have a side note really quick. I feel like geologists don't get enough credit for how cool their job is. Like you hear, I don't know,
22:23 astronauts, they're exploring something that we can't see. They're out of space. Same in the ocean, like you're exploring something, but like geologists don't get that same, like your job is so
22:34 cool. You're literally discovering things that we can't see and identifying it. And it's so important. It is, it is. Well, you know, and I'm glad to hear you say that because the industry is
22:47 being vilified in the media. And don't get me on my politics and don't start me down the road of climate
22:55 One thing as a geologist that you learn in school is you're studying the earth and you're studying the earth from the time It was formed up to where you are now. Right. So the earth has many
23:05 different cycles and it and we're constantly going through cycles The human lifespan if you if you look at the earth the existence of the earth as a 24-hour clock Humans have been on the earth the
23:19 last four seconds of the 24 hours
23:24 So our perception our understanding of the scale of the earth and what it's gone through in four and a half billion years Mm-hmm, and we've been around for four million years, but you know, that's
23:36 kind of the scale that you're looking at so for us to believe that oh my god, you know 30 years ago is this and 30 years from now it's gonna be this is is Probably not correct. Yeah, yeah, it's a
23:47 matter of scale. Just like anything else. Like reading an 8-point font or 72-point font. OK? Right. So - Well, I know you brought some slides for us. So for all of our listeners, make sure if
24:00 you have a chance. Check out the YouTube video. We will be sure to stitch them in so you guys know what we're talking about as we go through some. Mm-hmm. Let's - OK, so what I wanted to talk
24:13 about is machine learning. And there's different kinds of machine learning. There is the kind where you have
24:23 an endpoint here. And you want to get to an endpoint here. This is your solution. This is your beginning. And
24:47 so you bounce back and forth with machine learning in an algorithm, a mathematical algorithm, until a machine learning agrees with your conclusion. Now, that's not what I use I use a machine
24:47 learning called self-organizing maps, okay, which The first kind of machine learning is what they call supervised. You're supervising how it's working along and you make sure that it gets to your
24:55 endpoint, okay? What I'm using is called unsupervised. I don't know what's down on the ground. I want the computer to come back and tell me what's down there. People go in with preconceived ideas
25:08 as to what Mother Nature has. And it's like solving a puzzle. Trying to figure out geology is like solving a huge puzzle. And you're having to put all these pieces of information together in order
25:18 to hit bingo in order to get that last piece in and see the jigsaw fully complete. So I use a form of machine learning called unsupervised where there are no constraints. You send it down, you turn
25:34 it loose on a three dimensional seismic cube of the earth. And it comes back and tells you, here are all the patterns. What are you going to do with them? So the machine learning technology I'm
25:45 using, I've been using for about 12 years.
25:50 It was started, and I think I have a red, although red circles kind of moved a little bit, but self-organizing maps is what that red circle is supposed to be around, of what I focus on and where I
26:02 have found much success. Okay. Is, was started kind of by a gentleman by the name of Turi Tan, by the name of Tuvo Kehonen. So that kind of explains when your clientele look at this, they can
26:17 look at the different kinds of machine learning that are out there. The self-organizing maps is the best one in my mind when it's applied to seismic data. Okay. So if we go to the next slide, okay.
26:30 So,
26:32 generally by the name of Dr. Tuvo Kehonen
26:35 started playing around in the 80s with organizing information, okay, and doing it on the computer So if you were to imagine, say, you've got a file drawer
26:49 And the inside that file drawer is a bunch of folders. You have organized information into those folders. That is similar. So all your electric bills are in one folder, or all your water bills are
27:01 in a folder, or all your insurance payments are in a folder. So each folder contains similar information. Well, he started playing around with that back in the '80s. And he went to the UN And he
27:15 said, give me 39 different quality of life statistics - average age, average income, average education - on 130 some odd countries around the world. And he wanted to try to organize all that
27:33 information so that statistically, he could see the countries.
27:40 And so the next slide - and the first slide is the list of all the countries So the next slide, and I have to thank Dr. Tom Smith for this slide. is basically a picture of what we call the topology
27:54 or a self-organized map, a 2D map. That's the map of all the colors. And then you see the map of the world. And the map of the world is the different colors correspond to the colors in the 2D
28:08 color map. So you can see in yellow that the US and Canada have very similar statistical information about their population
28:18 And so what I'm doing in the seismic world is I'm doing the same thing. I'm breaking the seismic information down into colors or patterns. And then I can turn on and turn off any of those patterns
28:29 and see where in my seismic data those patterns exist. It's very simple, but it's very powerful to take. And you can do this in an Excel spreadsheet. Now, I don't know Excel well enough to put
28:41 the mathematics together, so I let the machine do it. But it started out as basically an Excel spreadsheet. And now it's to where it's part of a huge algorithm in software, and you say, read this
28:55 information, do your magic to it, organize the data, and then give me another volume in color with all the data organized. So this next example I have is how we're breaking it down. Okay, so
29:12 instead of looking, like I said earlier, the wiggle going this way and the wiggle going that way, we're breaking it down into very small time increments, which gives us statistically a lot more
29:22 information about the subsurface. So the next slide, and we're looking at different ways of breaking down the signal. So these are called seismic attributes. And in 1979, they took the recording,
29:38 the seismic recording, and they started splitting it out into different components Kind of like, kind of like taking a satellite picture
29:48 into various frequencies or rays or colors. I mean, we're seeing the total color spectrum with our eyes, but we know we can break that color spectrum down into blues, greens, yellows, and
30:01 oranges. And then colors we can't see. The same thing happens in sound, okay? You can have ultra high frequencies that you can't hear, but dogs can. Or then you have ultra low frequencies,
30:14 which you know rattle your whole body when you hear them So we're breaking down the sound signal into different components, different waves. And each one of those different components tells us a
30:25 little something different in the earth. So we call them seismic attributes. They're all split from the main seismic that was recorded, but we're just breaking it into different frequencies and
30:37 different signals. So this next slide is when it comes back to us, it comes back to us in the form of color And I've put a wiggle trace or a seismic trace. over it so you can see how much detail we
30:52 can see in the subsurface now that we couldn't see in the regular recording. And I think this first example at top, okay, is the seismic when we're on our workstations, the orange and black, and
31:06 people use different colors, but basically it's a range of orange, black and white, that's what we've interpreted for the last 50, 60 years, okay?
31:18 And we know that we can see hard rock and soft rock, but we can't see the details where there's a channel, the bottom of the channel looks like, what the top of the channel looks like. We can't
31:29 see all these different geologic features. Well now with the machine learning, that's the same picture just broken out into different statistical. Yeah. A lot more data. And so in this particular
31:42 example, I am tying to a well, and it's kind of hard to see there's a little red flag in the middle of that well where they perforated and produced oil. And that well made 400, 000 barrels of oil
31:56 in its lifetime. So a correct tie is to that yellow pattern, right? So if you go to the next slide, I can go in now that the yellow is actually
32:08 came out. Fuchsia.
32:11 So now I can go in just like the Kohana example and I can turn off all the patterns that I'm not interested in and just look at the pattern that I'm interested in. Then I can go through and say, oh,
32:24 that pattern produced it this well, that pattern produced it this well, that pattern produced this well. And oh, look, there's some patterns out here that have no wells in them. So maybe that's
32:33 a good target, right? So that's how I'm using the machine learning to help me and my clients find oil and gas.
32:43 Does that make sense? Yeah, it does. No, it is. When you put it in color, it's really good. It's really nice that we were looking at this before and we were like, Oh, what is that? What is
32:53 that? Yeah, so it's nice getting you to explain it all. Well, and so here's a good example. This next example, you've all heard of the Eagleford. All the shale plays are going on, right? Well,
33:04 in the main part of where
33:08 the Eagleford produces, you have what they call the Austin chalk, and then you have the Eagleford shale, and then below that, they have what they call the Buddha line. So the Austin chalk and the
33:17 Buddha are those big, hard pieces of earth that send a signal in one direction. It's called a peak. They're a peak of ends. And the poor little Eagleford is wedged between those two formations,
33:32 and so it ends up being the soft rock or the trough, okay? And where they're producing the Austin chalk, and again, it's in time, is about 30 milliseconds. So it's about 200 feet thick, not
33:45 very thick at all. And they're trying to drill wells for long distances, staying in that little piece. Now this slide is showing that the Austin chalk is broken out into six different layers,
33:60 which are impossible to see in regular seismic. But now we can break out the Austin chalk and we can see all six layers Probably the two darkest red layers in the middle are where most of the oil and
34:14 gas comes in the Austin chalk. And so people can't normally see that, but we can track it. So the next slide actually shows you everywhere in this map. And then we can go in with a client and
34:28 calibrate their well information. We can show them where they missed the sweet spot completely and they had a dry hole or a poor producer or where they have a really good producer because they,
34:39 their well, stayed vertical in that sweet spot. So this becomes very important in the shale plates as well, which before we could just see a trough and that's it. We couldn't break it out into its
34:53 components and know where the for the juicy part is. And so the next slide is really cool because here's a case where most of us would look at the seismic on the on the left hand side and just map
35:06 right through that low spot. Okay, that's just a dip. That's just a dip in the earth Well, the right hand slide is showing that that low spot actually is a channel cut and it's got different kind
35:19 of rock filled inside of it. Right. Okay. So if you're drilling a horizontal well, that's called a geo hazard. And you don't want to encounter that because it'll mess
35:32 that well, that would be called a geo hazard and we try to avoid them. Well, it happened. Well, it could mess up the well, it could mess up the mud system. I mean, they would get through it
35:41 But it's one of those things that you like to avoid. if you don't have the drill. But you couldn't see it. Right. You couldn't see it in the regular size mate. You wouldn't know that it's an in
35:50 size valley cut there. So that's how we're applying the technology. And it works in limestones, as well as it works in chales and sands. This next slide, I think, is an example. Yeah, that's
36:03 an example. So here's what we've interpreted for years and years and years. This is the top of the Edwards line. Okay, so the Edwards in Austin, Texas, is the aquifer. Okay. You know, you're
36:16 driving through Austin, you're going around 360 on the northwest side of Austin and, you say entering Edwards aquifer. Well, at 15, 000 feet, which is where this picture is taken from, it
36:29 carries gas.
36:32 And so this is a line that three wells have been drilled into this Edwards line.
36:43 one in the '70s and one in the '80s. Two of the wells
36:48 found good gas shows. But in the '70s and '80s, they weren't interested in natural gas 'cause the price was so cheap. They were looking for oil. So they ended up plugging the wells because even
37:00 though they had good gas shows, and then one well that was drilled in the
37:06 '70s, couldn't find anything. I mean, they didn't even have a whiff of gas So how do you tell where the reservoir is and where it's not when you just have one big orange line to look at? Right.
37:18 Right. Okay? Yeah. Next slide. Okay.
37:22 Wow. The machine learning. It's so much more. Well, what the machine learning does, and what's important here is you've got a big deep rock that's full of gas, and that rock is at 15, 000 feet
37:36 below the surface of the earth, and it's a limestone, so it's fractured, okay? And over time, little bit of that gas escapes into the rock above it. So what the machine learning is designed to
37:49 do is look for subtle changes in rock properties of the subsurface. And you can see I've highlighted kind of a blue area there. Okay. That is a signal where the gases escape into the shell above
38:03 the Edwards. Wow. So everywhere you see that blue, it's being charged from that pressure gas below it in the rock. So the next line will actually show you all three wells. And where the blue is,
38:18 is where you've got good porosity and good reservoir. And where the drywall is. Yeah. Well, the next slide after that, I feel like that would be very frustrating as the whoever drilled that like
38:29 you're just off. Yeah. Well, you're going to find out how just off. Okay. So here's, here's a what they what we call a dip line. This is the Edwards. It's a reef. It's actually an old buried
38:40 reef. Wow. And that's why the structure looks like that. So if you look at where that well is drilled in conventional seismic, you can't see why it was a dry hole. But if you look at the blue and
38:51 green and that well is not in the blue or green, that's where it was tight. Okay. It's in kind of an orgy yellowy stuff, right? If they had moved that well, 400 feet north,
39:05 they would have had a discovery. I feel like I would throw. I know I'd be like 400 feet. Well, they didn't even have the 3D in this area when they drilled those well. Yeah. This 3D was shot in
39:18 the mid 90s. Okay. So the next slide. Okay. So this is the, this is the major oil company. Well, that was drilled in the 60s. It had all the shows. Okay. But then the 60s gas was really
39:28 cheap and they didn't, they were looking for oil. They didn't want gas. So they walked away from this well. If they had drilled 400 feet south, they would have missed it entirely So here you have
39:40 a dry hole that missed it by 400 feet. you have a well-to-head good shows that miss being a dry hold by 400 feet. It's real wild cat and vet things. Yeah. But that's just to show you how the
39:52 technology has changed the way we interpret what's in the subsurface. Yeah. Do you feel like the majority of companies nowadays are using the shema? No. No.
40:04 How are they? No. Yeah. Well, I asked that myself all the time, which as a consultant is one reason why I stay really busy. Right. Because because when companies see what this can do for them,
40:16 then they say, well, I don't want to take the time to learn how to do it. I'll just get Deborah. Yeah. So I stay. I mean, yeah. Well, it's good for me, except, you know, as I'm getting
40:26 older, maybe not work as much. But but I have a I have a huge backlog of clientele. Right. That have me doing this, especially the little guys. Maybe they only have one or two 3Ds. Now the big
40:36 companies, Chels, Exxon's, they have research departments. Right. And I'm
40:42 that they're working on in the research department. But what I've found over the years with the big companies is that a lot of time it never makes it out of research into the hands of people who
40:51 could actually use them. Right, because they're gatekeeping. They're gatekeeping. It's their little world. So anyway, and I think there's one more example in here where now we have machine
41:04 learning that can automatically pick breaks in the earth. We call them faults and around salt domes, salt domes are the slow growth and pushing up of salt into rock. And as it pushes through, as
41:18 it pushes its way through the rock, it fractures the rock, tends to be
41:25 a radial type pattern around the top of the dome. So we can use machine learning instead of hand-picking all the faults, which, you know, compartmentalizes the oil and gas. Different fault blocks
41:36 may have oil and gas at different levels. But now we can do, let the machine do it. And the next slide shows an example of, this is a project I got and a consultant had worked on it for years,
41:52 picking, hand picking all the breaks in the earth that he saw. And he gave me the project that had 280 different hand picked breaks of the earth, okay, hundreds of man hours to do that Of course,
42:07 he's being paid by the hour, so he probably didn't bother him at all. I was able to do the same work in four hours on my machine by letting the machine do it. Amazing. So, that's just an example
42:20 of how I'm using this to find oil and gas. I feel like this is going to be a loaded question, but as we wrap up, I think I definitely want to know, obviously, it's come a long way over the last
42:30 few years So, next five to ten years, where do you see this even, you know, progressing even further? Well, of course, now we have. dimensional seismic. Okay. What does that even mean? Okay.
42:43 So what's the fourth dimension? Well, time is the fourth dimension. Okay. So, so you have you, you, you put your recording devices out over a big field that you discovered. And you're
42:56 producing from this field. And you just leave the recording devices out there. And every six months or so, you shoot a brand new 3D And so, over time, you can see the depletion of the oil and gas
43:08 from the rocks. So that's four dimensional. And I think that'll come into play more. And certainly, I see advances in the machine learning aspect to where it becomes a household name. I mean, it
43:22 took a long time for people to get used to going from 2D to 3D
43:28 mainly because of cost and technology. But now, the technology for recording and processing and getting it into your hands, that's all gotten cheaper. and faster. So the same thing's going to
43:42 happen with the machine learning and AI. I think that's that's our next revolution. Like I said earlier, the easy stuff has been found. And
43:50 so we if we're going to keep on finding oil and gas and keeping the earth sustained and energy, then we're going to have to use better and better tools for sure. Yep. So we always end each episode
44:03 with three rapid-fire questions, Misty. Sure.
44:08 What in your opinion is the biggest misconception in the energy industry?
44:19 And you know, I think the biggest misconception in the energy industry is that we're destroying the earth.
44:27 If you look at the lithium mines and the cobalt mines and the rare earth minerals that they're digging up and creating these huge
44:38 cuts in the earth, you get minerals to get rare earth minerals to make batteries and using slave labor for that? I didn't want to get in there, yeah. And you look at, you look at that compared to
44:51 drilling one natural gas well. And one good natural gas well can supply all the electricity that it would take to run 10, 000 cars or 20, 000 cars or more So I think the idea that we're ravaging
45:08 and pillaging the earth is not being translated very well. Right. I think there needs to be more education around where absolutelys are coming. Right. Right. 100. Well, one of the things that
45:19 scares me is that we don't have enough students that are in STEM systems. We don't have enough math, we don't have enough science being taught in the school. And for a long time, geology or earth
45:30 science was part of the curriculum. It's been removed. So now - Yeah, so except in a few schools, districts, so, so. kids don't have a chance to learn about the earth and what goes on below it.
45:45 So they don't have an appreciation for what we're doing. Right, yeah, that's sad.
45:53 Why should people care about energy?
45:58 Well, I mean, it keeps us alive for fun. Most importantly, we would be dragging knuckles in the sand and, you know, hoping lightning would strike a forest fire So we can cook some meat. I mean,
46:11 it's very, very animal. Yeah, well, I'm a hunter, so yeah. But no, I mean, energy is, and here's another misconception. People don't understand that everything they use comes in some way or
46:26 form from petroleum. We talk about it all the time. To the mask area you put on your eyelashes at a lipstick, to, you know, the plastic that makes up the frames on your glasses If you took away
46:38 all the petroleum products. would be dragging your knuckles in the sand. Right. But naked. Right? Because you would not close. The third one is the fun one. Yes. In your career, what is the
46:52 most embarrassing story? Or what is your most embarrassing story?
46:58 Well, I try not to embarrass myself Kind of think, you know, of 47 years in the industry. I've had some embarrassing moments, that's for darn sure. I think I was giving a presentation one time
47:13 instead of very bad word.
47:16 Because something went wrong with the projection system. Back in the days when we had little slide. Yeah. And we had a carousel. Yeah. They get stuck or something like that. It's a time
47:25 presentation and you're trying to get through everything. And sometimes just bad things slip out. Uh-huh. I get it.
47:33 We couldn't edit live programming. Right. Exactly. That's funny. Well, thank you so much for coming on. This was honestly so - So knowledgeable. Informative and I feel like I learned so much
47:49 and can now look at your slides and be like, okay, that makes business. Yeah, I love that. We should have recorded ourselves trying to look at
47:58 that. We definitely should have - It was bad. Maybe we should record Jules. Yeah. We could get her crystals. We'll talk a lot of everyone in our office. Well, now that you look at it, then you
48:05 can see what's going on. There's one more slide we didn't get to. Oh So
48:10 I take the fault information, and I can combine it with the other attributes to see faulting and one swoop. So now I can see the stratigraphic layers along with where the earth is broken.
48:27 That's the thing. That is. So now that you understand how the slides work, then you can go over and look at them and stuff like that. Yeah, that's awesome. Where can people find you if they want
48:37 to learn more Can I - LinkedIn? Well, yeah, I'm on LinkedIn. That's kind of how I found you guys, actually. And I've had the same email, can believe it or not, for 30 years. It's D, Sacri,
48:51 S-A-C-R-E-Y, at AuburnEnergycom. All one word. We can put that in the show now, so - We will. Yeah. All the things that people can - All right. I don't like texting, you know, I'm still the
49:01 old-fashioned kind. That's okay. I don't wear my thumbs out. But certainly email, I respond to you very well. Okay. Okay Thank you again. You're welcome. Come back and miss anytime. This was
49:11 fun, absolutely. Well, you just tell me what next topic you want, and then I'll come up with something. Yeah, we need to get you on the, what's it called, energy bites. Ooh. That would be a
49:21 good data one. Yeah. Sure, sure. All right. Like and subscribe.
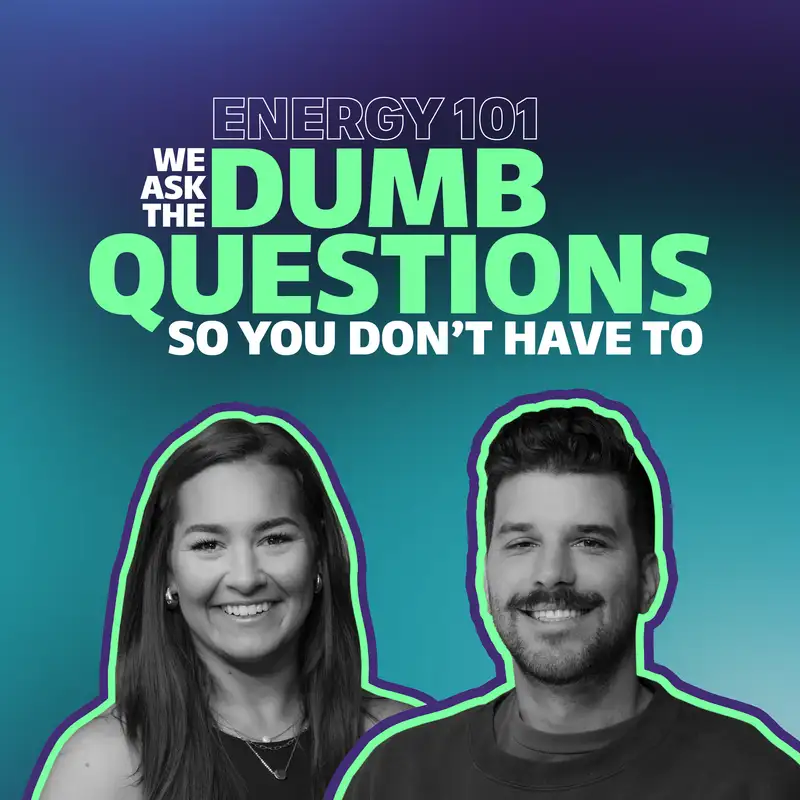